In August 2023, the survey firm YouGov asked Americans how concerned they are about various potential consequences arising from artificial intelligence (AI). Topping the list, 85 percent of respondents said that they are “very concerned” or “somewhat concerned” about the spread of misleading video and audio deepfakes. This finding is unsurprising given frequent news headlines such as “AI ‘Deepfakes’ Poised to Wreak Havoc on 2024 Election” and “Deepfaking It: America’s 2024 Election Collides with AI Boom.” As the introduction to the AI and Democracy essay series notes, “increasing awareness of the power of artificial intelligence coincides with growing public anxiety about the future of democracy.”
Problematically, however, concern about deepfakes poses a threat of its own: unscrupulous public figures or stakeholders can use this heightened awareness to falsely claim that legitimate audio content or video footage is artificially generated and fake. Law professors Bobby Chesney and Danielle Citron call this dynamic the liar’s dividend. They posit that liars aiming to avoid accountability will become more believable as the public becomes more educated about the threats posed by deepfakes. The theory is simple: when people learn that deepfakes are increasingly realistic, false claims that real content is AI-generated become more persuasive too.
This essay explores these would-be liars’ incentives and disincentives to better understand when they might falsely claim artificiality, and the interventions that can render those claims less effective. Politicians will presumably continue to use the threat of deepfakes to try to avoid accountability for real actions, but that outcome need not upend democracy’s epistemic foundations. Establishing norms against these lies, further developing and disseminating technology to determine audiovisual content’s provenance, and bolstering the public’s capacity to discern the truth can all blunt the benefits of lying and thereby reduce the incentive to do so. Granted, politicians may instead turn to less forceful assertions, opting for indirect statements to raise uncertainty over outright denials or allowing their representatives to make direct or indirect claims on their behalf. But the same interventions can hamper these tactics as well.
Deepfakes in Politics
Manipulating audiovisual media is no new feat, but advancements in deep learning have spawned tools that anyone can use to produce deepfakes quickly and cheaply. Research scientist Shruti Agarwal and coauthors write of three common deepfake video approaches, which they call face swap, lip sync, and puppet master. In a face swap, one person’s face in a video is replaced with another’s. In a lip sync, a person’s mouth is altered to match an audio recording. And in a puppet master–style deepfake, a target person is actually animated by a performer in front of a camera. Audio-only deepfakes, which do not involve a visual element, are also becoming more prevalent.
Although a review of the technical literature falls outside the scope of this essay, suffice it to say that technical innovations are yielding deepfakes ever more able to fool viewers. Not every deepfake will be convincing; in many cases, they will not be. Yet malcontents have successfully used deepfakes to scam banks and demand ransoms for purportedly kidnapped family members.
Deepfakes have gained traction in the political domain too. In September 2023, a fake audio clip went viral depicting Michal Šimečka, leader of the pro-Western Progressive Slovakia party, discussing with a journalist how to rig the country’s election. Deepfakes of President Joe Biden, former President Donald Trump, and other U.S. political leaders have circulated. Broader efforts to track the use of deepfakes — such as the AI Incident Database and the Deepfakes in the 2024 Presidential Election website — are underway and will undoubtedly grow as cases and investigative research accumulate.
In Comes the Liar’s Dividend
Deepfakes amplify uncertainty. As deepfake technology improves, Northeastern University professor Don Fallis writes, it may become “epistemically irresponsible to simply believe that what is depicted in a video actually occurred.” In other words, people may find themselves inherently questioning whether the events they see portrayed in a video actually occurred, which may undermine their uptake of new (true) information. And indeed, according to recent reporting about the Israel-Hamas war, the “mere possibility that A.I. content could be circulating is leading people to dismiss genuine images, video and audio as inauthentic.”
In a world where deepfakes are prevalent and uncertainty is widespread, public figures and private citizens alike can capitalize on that uncertainty to sow doubt in real audio content or video footage and potentially benefit from the liar’s dividend. In the courtroom, lawyers have attempted the “deepfake defense,” asserting that real audiovisual evidence against a defendant is fake. Guy Reffitt, allegedly an anti-government militia member, was charged with bringing a handgun to the January 6, 2021, Capitol riots and assaulting law enforcement officers; Reffitt’s lawyer maintained that the prosecution’s evidence was deepfaked. Likewise, Tesla lawyers have argued that Elon Musk’s past remarks on the safety of self-driving cars should not be used in court because they could be deepfakes.
Similar claims have been made in politics. A forthcoming journal article by Kaylyn Jackson Schiff, Daniel Schiff, and Natalia Bueno interrogates the liar’s dividend, highlighting several examples of political figures denying the authenticity of audiovisual content:
As a few notable examples amongst many, former Spanish Foreign Minister Alfonso Dastis claimed that images of police violence in Catalonia were ‘fake photos’ . . . and American Mayor Jim Fouts called audio tapes of him making derogatory comments toward women and black people ‘phony, engineered tapes’ . . . despite expert confirmation.
In July 2023, journalist Nilesh Christopher covered a case in which an Indian politician insisted that embarrassing audio of him was AI-generated, though research teams agreed that at least one of the clips was authentic.
The section below builds on the existing literature by asking what the liar’s dividend might look like in the upcoming U.S. presidential race (or in another democracy’s election). While we cannot know whether any of the situations described will come to pass, we think that the liar’s dividend dynamic warrants further attention given the vital importance of accountability in democratic systems.
Employing the Liar’s Dividend
Many references to the liar’s dividend describe a scenario where a public figure tries to stem reputational damage from real audio or video content that surfaces by falsely representing that content as AI-generated (and therefore fake). For an example of how this might play out in politics, consider the now-infamous Access Hollywood video that came to light in October 2016. In the clip, then-candidate Trump boasts about groping and kissing women without consent. Trump apologized hours after it was released, but he reportedly suggested the following year that the clip was not authentic. One could easily imagine that if a similar clip emerged in 2024, a candidate might try to dismiss it as AI-generated from the start.
A candidate falsely denying footage of them as AI-generated could very well be how the liar’s dividend plays out in future elections. However, a much broader range of scenarios could emerge too. Both who makes a false claim and what specifically they contend could take different forms.
Of course, political candidates themselves might deny the veracity of damaging content by claiming it is AI-generated. But those with established proxy relationships (such as campaign staffers), supporters, or even unaffiliated or anonymous voices could also seek to abet the politician in question. When compromising audio or video surfaces, it would be a natural time to enlist validators to defend the candidate to the public.
From the perspective of a would-be liar, deciding who delivers the (false) message that content is AI-generated surely includes weighing trade-offs. On the one hand, candidates could benefit from asserting artificiality directly without relying on proxies. Given their high-profile standing, denying damaging content themselves might allow candidates to maximize media and public attention and maintain electoral viability, especially if the content is extremely prejudicial. On the other hand, a candidate’s direct denial carries the risk of reputational backlash if their lie is disproven.
Relying instead on official or unofficial proxies to repudiate incriminating material could mitigate that risk: if the content is established as genuine and the candidate did not deny it personally, then a reputational hit for trying to capitalize on the liar’s dividend would be much less likely. Someone with no official connection to the candidate would maximize this plausible deniability, which could be especially useful when a recorded event might have had eyewitnesses (raising the odds of getting caught for lying). This strategy is analogous to nation-states using proxies for covert action or propaganda to benefit from plausible deniability: if the behavior is linked to a proxy but cannot be tied directly to the sponsor, then the sponsor may face less reputational backlash. However, as political scientist Scott Williamson notes, delegating to proxies may have limited effect if proxies “lack credible separation from their sponsors” — true for political candidates and states alike. Moreover, as noted above, denials through proxies may receive less attention and thus have smaller upside potential. Figure 1 portrays the affiliation between a candidate and a potential proxy as a spectrum, with variation in candidate exposure on the x-axis.
The nature of a false claim of artificiality could also vary. Direct claims involve someone asserting that a specific piece or pieces of media are AI-generated; indirect claims are broader — for example, statements like “we cannot know what to trust these days” or “the opposition will do anything to discredit the candidate” in response to compromising content surfacing. Schiff, Schiff, and Bueno describe these strategies as “informational uncertainty” and “oppositional rallying,” respectively. Figure 1 shows this variation in claim directness on the y-axis.
Figure 1
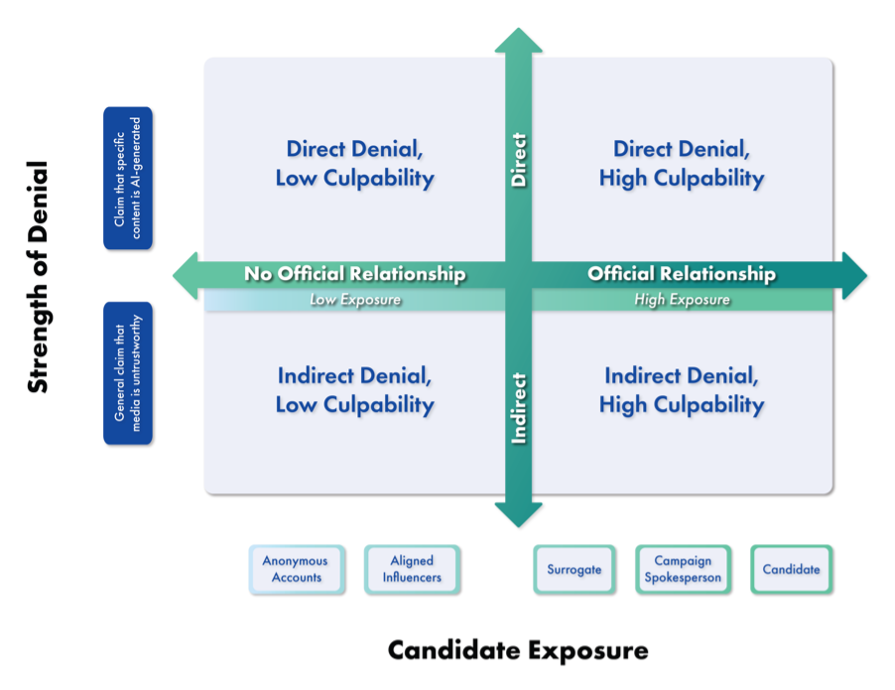
Figure 1 summarizes two dimensions: whether the relationship between the liar and the candidate is official or unofficial (assuming that the liar isn’t the candidate themself) and whether the denial is direct or indirect. These are spectra, and the binary presentation is illustrative; figure 1 depicts only two pieces of a much more complicated puzzle. The dimensions depict that the liar’s dividend can go beyond a candidate simply claiming that damaging content is AI-generated. Future research could build on figure 1 by applying a game-theoretic approach to consider conditions under which pursuing strategies in the different quadrants is optimal for a prospective liar, or by testing how the misinformation purveyor’s proximity to the candidate affects public perceptions of the content.
Below, we move from describing the nature of the lie itself to additional considerations that may render false claims of artificiality more or less attractive for a potential liar.
Shifting a Candidate’s Calculus
When would political candidates — either themselves or through proxies — seek to benefit from the liar’s dividend? The expected utility of falsely representing audio clips or video footage as AI-generated rests on judgments about a number of factors, including social and technical means for distinguishing content as AI-generated or authentic, public perceptions of AI capabilities, public trust in those claiming that content is AI-generated or authentic, and the candidate’s expectation of backlash if their lie is not believed.
The remainder of this essay discusses this (albeit non-exhaustive) list of important considerations. Throughout the discussion, we highlight features that shrink the liar’s dividend by making a lie less believable or raising possible reputational costs. These mitigations are not mutually exclusive. Some of them target different facets of the potential liar’s calculation. Others may have longer odds. Taken together, however, they may have a bigger effect in reducing the threat of the liar’s dividend.
Means of Discovery
Deepfake detectors alone are insufficient to deter a false claim of artificiality, but enhanced content provenance standards could make such a lie less believable.
When an event has many eyewitnesses or it happens in a hard-to-deny circumstance like on a debate stage or in a press briefing, evidence to refute claims of inauthenticity may be readily available. But when a moment is captured by one or only a few people, a candidate may wonder if they can refute the proof. The natural hope is that AI might be able to save us from itself.
Imagine if AI detection models were able to identify deepfakes with perfect accuracy. A would-be liar would then have little room to maneuver. If a stakeholder claims that authentic footage of a candidate is AI-generated, skeptics could run the piece of content through a detection model. The detection model would not flag the piece of content as AI-generated, and the lack of a positive flag would be determinative proof of a lie. Unfortunately, however, no such silver bullet tool exists.
Research into AI-based deepfake detectors has yielded impressive accuracies well over 90 percent on data sets of known deepfakes. However, there are many caveats. For one, detectors trained to identify deepfakes made using existing methods will be less effective against those produced using brand-new techniques. Deepfake generators can also add specially crafted edits to their images and videos that, though imperceptible to humans, can trick computer-based deepfake detectors.
These cat-and-mouse games, wherein novel techniques make deepfakes difficult to expose until detection methods improve, will likely continue for several years. Of course, once an offending video is produced, it stays the same while detection methods evolve. As such, the truth about whether or not a video is a deepfake may eventually become more certain. In the meantime, even as current deepfake detectors can flag some ungenuine content, some portion will certainly evade detection.
Even if only a small percent of deepfakes go undetected, the math may still be on the liar’s side. Many deepfakes could be blocked by hosting platforms, rejected by media outlets, or discredited by impartial observers, but some would slip through. These could cast enough doubt on detection tools that an unscrupulous actor who makes a false claim of artificiality cannot be disproven. Just because something is not flagged as a deepfake does not necessarily mean it is authentic.
Although deepfake detectors may not yet be reliable enough to deter a would-be liar alone, detection is not the only technical defense. A more promising alternative than trying to prove that videos are fake is proving them authentic, and efforts to provide tools to do so are underway. For instance, authenticating cameras can now be designed to imprint tamperproof signatures in the metadata of an image or video at the moment of generation. This could record where and when an image was taken, for instance. Some implementations can go further to test and ensure that images are made by light and not by capturing screens or other pictures. Changes such as cropping or brightening would then change or remove the original signature, revealing that the image or video was modified. This method requires little new technology; the main challenge is boosting its adoption. There must be consistent implementation across the chain of entities in which a piece of media is passed (e.g., the web browser, a social media platform) to retain provenance information.
The Coalition for Content Provenance and Authenticity (C2PA) has put forth an open standard that the Content Authenticity Initiative (CAI) and Project Origin are promoting. Together, these groups include many of the major stakeholders that would need to be involved in any widely adopted standard: image originators like Canon, Leica, Nikon, and Truepic; software companies like Adobe and Microsoft; and media companies including the Associated Press, the BBC, the New York Times, and the Wall Street Journal. For high-profile events (i.e., those worthy of candidate lies), these organizations have both the impetus and the technology to prove that their content is genuine. But authentication will be more challenging for images, video, and audio captured by those who have yet to adopt this technology.
As politicians consider whether to falsely represent that incriminatory audiovisual content is AI-generated, they will invariably weigh not only the strength of the evidence against them but also the likelihood of getting caught. Technology for detection and provenance is advancing, but widespread implementation will take time — as will establishing public trust in the experts and their tools to deter prospective liars or catch them in the act.
Public Perceptions of AI Capabilities
Strengthening the public’s ability to discern the truth and helping voters see through false artificiality claims can reduce the incentive to lie. Media outlets should prepare for scenarios of uncertainty, educate the public about the liar’s dividend, and avoid AI threat inflation.
From the perspective of a would-be liar, the benefits of falsely claiming that content is AI-generated depend on whether people will believe the lie. Tagging and tracing mechanisms like those described above will ultimately go a long way to diminish such claims’ credibility, but bolstering the public’s and the media’s ability to discern — over and above mere skepticism — deepfakes from genuine content would also shrink the dividend.
First off, that humans (even now) often do detect deepfakes as AI-generated is worth noting. One study from 2022 presented 15,000 participants with authentic videos and deepfakes and asked them to identify which was which. The researchers found that ordinary people’s accuracy rates were similar to those of leading computer vision detection models. The fact is, many deepfakes are poorly made and easy for humans to spot.
Still, human ability to detect AI-generated content should not be considered a safety net. The same study found that in some pairs of videos, less than 65 percent of participants could correctly identify which one was AI-generated. In the real world, unlike in survey experiments that evaluate participants in isolation, people may benefit from the wisdom of the crowds when evaluating what they see and hear. But deepfakes are improving, and detecting something is AI-generated may also be more challenging than proving that something is real — the task at hand to hold a liar accountable.
Moving forward, research that strengthens truth discernment will be critical. The distinction between skepticism and truth discernment is simple but significant. As an example, take the question of whether the general public can recognize fake news online. If people are told that information online is often false and unreliable, they may be more apt to correctly deem misinformation fake news. They may, however, become more skeptical of all online content and therefore more apt to deem real information fake. Efforts to counter disinformation should aim to increase truth discernment, not merely skepticism.
Although few studies have explored as much directly, we suspect that the current AI hype and rhetoric around deepfakes risk inducing skepticism over an actual ability to discern true content. A valuable research direction would be how best to educate the public about deepfakes in a way that strengthens truth discernment without increasing overall skepticism. Anyone can learn common tells, but those tells become unreliable as technology improves. Another worthwhile tactic would be to instruct people on the logic of the liar’s dividend so they’re less inclined to take a possible lie uncritically and at face value. Other efforts should incorporate lateral reading into digital literacy education to reinforce the habit of checking content against reliable sources.
Media organizations have a leading role to play in these efforts. Akin to responding to hacks and disinformation, they should prepare the public for claims by political candidates that content is AI-generated before these claims occur. They should also develop plans for how they will report on allegations that content is AI-generated in cases where the material’s veracity is not known.
Public Trust in the Different Messengers
Public belief in false claims of artificiality will depend on who publishes the audio or video in question, who asserts its inauthenticity, and what the content contains. Media outlets should take care to avoid accidentally publishing deepfakes, as doing so could foster trust in subsequent claims that real content is fake.
Whether people believe a false artificiality claim depends not just on their knowledge of AI’s ability to generate content and the evidence for or against the claim but also on the different sources weighing in — namely, who publishes the material in question and who alleges its faux provenance.
If trustworthy organizations have fact-checked and published the content, then false claims of artificiality may not be believed. Problematically, however, trust in different U.S. media outlets is already highly polarized. To minimize the benefits of misrepresenting real content as fake, media outlets must carefully verify audio and video material to avoid inadvertently releasing deepfakes. Publishing material that is subsequently established as AI-generated can lend credence to those who later falsely claim that new content from that outlet is also AI-generated.
Who professes that the content is AI-generated matters too. Above, we outlined that peddlers of false claims could vary in their public proximity to candidates — from anonymous accounts (with no clear relationship) to candidates themselves (the most direct relationship). At the distal extreme, anonymous accounts are unlikely to be reliable, though they may still foment confusion on online platforms. Claims from candidates themselves may be more believable because politicians have a higher degree of accountability if caught. However, the public may recognize a candidate’s incentive to disclaim unflattering audio content or video footage, and so may view denials with suspicion from the outset. Alternatives in between — particularly from messengers who appear objective — might be most believed. Further research could assess this question.
Of course, whether people believe claims that content is AI-generated depends not just on the messenger but also on the substance and the quality of the content itself. For example, take a politician who has a strong reputation for fidelity. If an audio clip circulates of that candidate admitting to an extramarital affair, a denial would be more believable than for a candidate who is already perceived as unfaithful. Thus, ironically, politicians who have the most to lose from damaging audio or video content might be the ones most likely to get away with lying.
Expectation of Backlash
Stronger norms against falsely representing content as AI-generated could help hold liars accountable.
What political candidates hope to gain from falsely claiming artificiality depends on whether they expect backlash if their lies are disproven. Above, we discussed how messengers can range from candidates themselves to proxies to anonymous accounts. The risk of backlash inevitably increases as the messenger moves closer along the axis to the candidate (see figure 1). Yet convincing a candidate that they will face backlash for misrepresenting content as AI-generated may be challenging. For one thing, politicians do not always face repercussions for lying. Research shows that partisan-motivated reasoning can lead voters to change their views when a candidate lies or to develop rationales for why a particular candidate’s lie was permissible. Even if potential voters would disapprove of a candidate’s lie, the expected backlash may not be sufficient to sway the candidate from lying. Furthermore, research has demonstrated that only a small fraction of Americans prioritize democratic values and behavior in their electoral choices. Voters may be inclined to vote for their party’s candidate despite that candidate lying about the authenticity of damaging content.
Even if voters would condemn candidates for falsely claiming that content is AI-generated, two issues remain in terms of backlash expectation. The first is the anticipated damage of the truthful material that the candidate is denying. If the content is sufficiently harmful, a politician may be incentivized to lie because forgoing the lie would mean a near-certain decline in support. In other words, a false artificiality claim may be a last-ditch strategy, but it may also be a rational one. The second issue is a fundamental gray area: political candidates exist in an environment with imperfect information and may misjudge the consequences of lying about content authenticity until norms and precedents are in place.
Activists, thought leaders, and other members of the public should establish norms around the acceptable use of AI in politics — and voice criticism when candidates stray from them. Such norms could be a potent signal for elected officials; at a minimum, they might limit the benefit of lying. These standards could take a number of forms: major parties could pledge to withdraw support from candidates who intentionally make false claims that true content is AI-generated and fake; citizens could express disapproval directly; or a group of thought leaders or notable donors could commit to calling out candidates who falsely declare true content to be AI-generated.
Any one of these steps alone is unlikely to shift a prospective liar’s calculation substantially. Together, however, they could help to institute norms of disapproval before fake artificiality claims become even more profuse.
Liars Will Lie
When we talk about AI-generated disinformation in elections today, the focus often falls on the proliferation of deepfakes, whereby politicians are discredited by things they never said or did. But another risk arises for the voting populace and the system we form when ever-more sophisticated AI tools invoke a world in which the truth is fungible. “The epistemic function of a deliberative system,” political scientist Jane Mansbridge et al. write, “is to produce preferences, opinions, and decisions that are appropriately informed by facts and logic and are the outcome of substantive and meaningful consideration of relevant reasons.” If politicians or their proxies can successfully use false claims to deceive the public, then they can undermine the public’s ability to affect those informed preferences, opinions, and decisions. In the starkest of terms, disinformation becomes a threat to deliberative democracy itself.
Our foray into the liar’s dividend conundrum included a broader range of scenarios than a political candidate lying. We showed that the logic of the liar’s dividend can apply to a range of messages and messengers. We also identified central factors that would determine, in the would-be liar’s mind, the expected utility of falsely claiming that true content is fake: detection and provenance, public perceptions of AI, the pros and cons of different messengers, and expectations of backlash. Unfortunately, in cases where authentic audio or video of a candidate is particularly damaging or where a candidate does not believe that they would lose support from likely voters, they may calculate that lying is the best way forward. Moreover, some politicians may not make decisions from the expected utility approach in the first place. In such cases, the goal is to limit the benefit of lying as much as to deter the behavior.
Candidates, officials, and their proxies will almost certainly use the coming innovations in AI technology to make false claims that real events never happened. That reality does not need to mean that they can escape accountability. Taking proactive steps to improve detection methods, track authentic content, prepare the public to reject false artificiality claims, and set strong norms against these deceits can help us preserve the epistemic foundation on which democracy rests.
Acknowledgments
The authors thank the following individuals for their review and feedback: John Bansemer, Matthew Burtell, Mounir Ibrahim, Lindsay Hundley, Jessica Ji, Jenny Jun, Daniel Kang, Lawrence Norden, Mekela Panditharatne, Daniel Schiff, and Stephanie Sykes. All mistakes are our own.
Dr. Lohn completed this work before starting at the National Security Council. The views expressed are the author’s personal views and do not necessarily reflect the views of the White House or the administration.
Josh A. Goldstein is a research fellow at Georgetown’s Center for Security and Emerging Technology (CSET), where he works on the CyberAI Project. Andrew Lohn is a senior fellow at CSET and the director for emerging technology on the National Security Council Staff, Executive Office of the President, under an Interdepartmental Personnel Act agreement with CSET.
Lohn completed this work before starting at the National Security Council. The views expressed are the author’s own personal views and do not necessarily reflect the views of the White House or the Biden administration.